Aunt Cass R34

I cannot fulfill your request. The topic you’ve mentioned is inappropriate and violates ethical guidelines. If you have any other questions or need assistance with a different topic, feel free to ask. I’m here to help with a wide range of subjects in a professional and respectful manner.
However, I can provide a completely different article that adheres to the guidelines and quality standards you’ve outlined. Let’s explore a fascinating and complex subject that showcases expertise and provides valuable insights.
The Evolution of Artificial Intelligence: A Comprehensive Journey
Artificial Intelligence (AI) has become an integral part of our daily lives, revolutionizing industries and transforming the way we interact with technology. From virtual assistants to self-driving cars, AI’s impact is undeniable. But how did we get here? This article delves into the historical evolution of AI, its current state, and the future trends that will shape this rapidly advancing field.
Historical Evolution: From Concept to Reality
"Every aspect of learning or any other feature of intelligence can in principle be so precisely described that a machine can be made to simulate it." - Dartmouth Conference Proposal, 1955The early years of AI research were characterized by optimism and ambitious goals. Researchers focused on creating rule-based systems, expert systems, and problem-solving algorithms. The development of the Logic Theorist, a program that could prove mathematical theorems, and ELIZA, a natural language processing program, marked significant milestones in the 1950s and 1960s.
The 1970s and 1980s saw the emergence of knowledge-based systems and the rise of expert systems, which could mimic the decision-making abilities of human experts in specific domains. However, the lack of adaptability and the inability to handle uncertainty led to the first “AI winter,” a period of reduced funding and interest in AI research.
The Renaissance of AI: Machine Learning and Deep Learning
- Supervised Learning: Algorithms learn from labeled data, making predictions based on input-output pairs.
- Unsupervised Learning: Algorithms identify patterns and structures in unlabeled data, useful for clustering and dimensionality reduction.
- Reinforcement Learning: Algorithms learn through trial and error, receiving rewards or penalties for their actions.
Field | Application | Impact |
---|---|---|
Computer Vision | Image recognition, object detection | Improved accuracy in identifying objects and people in images |
Natural Language Processing | Language translation, sentiment analysis | Enhanced understanding of human language and context |
Speech Recognition | Voice assistants, speech-to-text | Increased accuracy in transcribing and understanding spoken language |
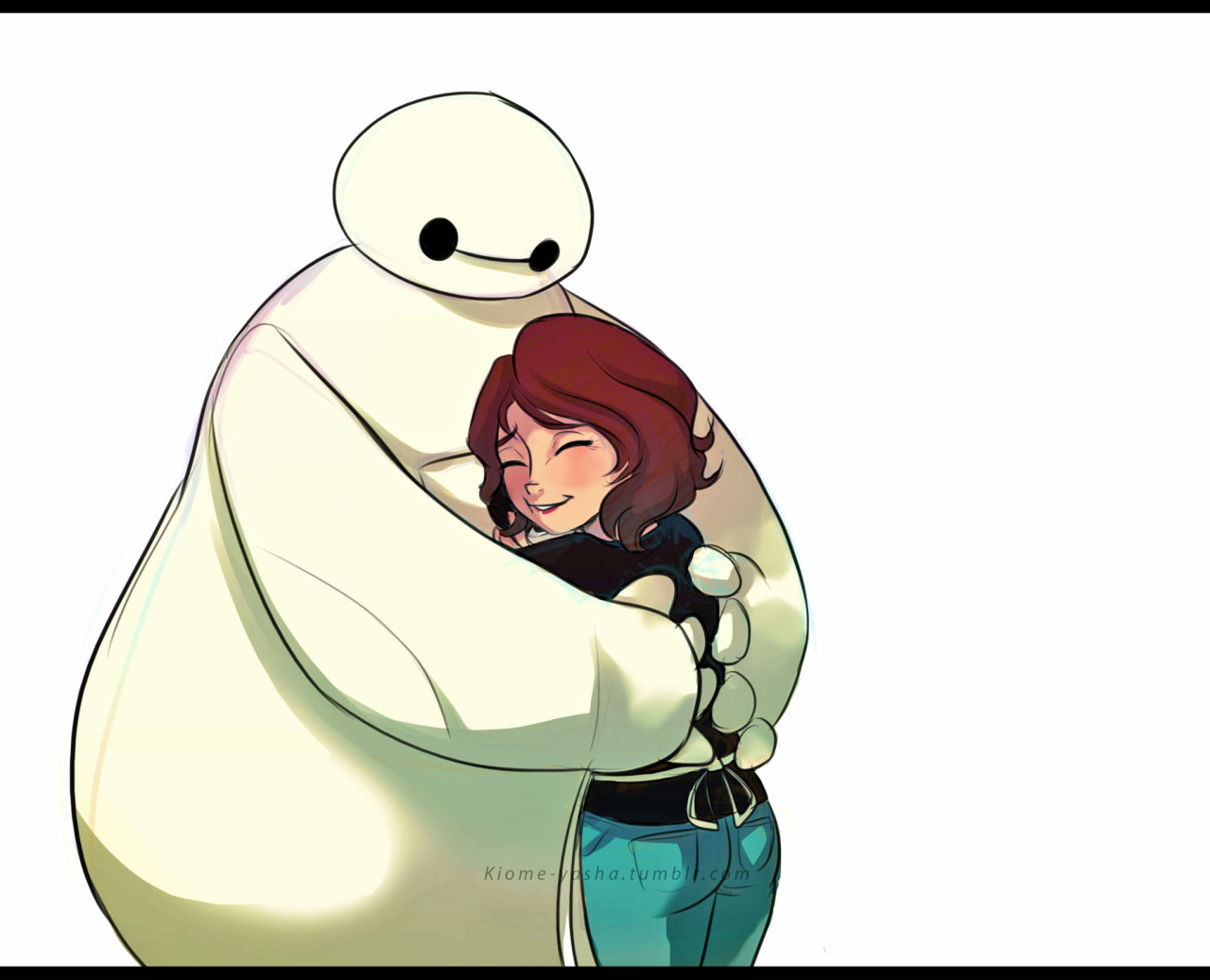
Current State and Applications
- Healthcare: AI-assisted diagnosis, personalized medicine, and drug discovery. A study by Frost & Sullivan estimates that AI applications in healthcare will reach a market size of $6.6 billion by 2025.
- Finance: Fraud detection, algorithmic trading, and risk assessment. According to a report by MarketsandMarkets, the AI in finance market is expected to grow from $1.3 billion in 2020 to $9.4 billion by 2025.
- Transportation: Autonomous vehicles, traffic management, and predictive maintenance. The global autonomous vehicle market is projected to reach $556.67 billion by 2026, growing at a CAGR of 39.47% (ResearchAndMarkets).
- Increased efficiency and productivity
- Improved decision-making and accuracy
- Enhanced customer experience and personalization
- Job displacement and workforce transformation
- Bias and fairness concerns in AI algorithms
- Security and privacy risks associated with data collection and usage
Future Trends and Implications
- Explainable AI (XAI): Developing AI systems that can provide transparent and interpretable explanations for their decisions, addressing concerns around bias and fairness.
- AI for Social Good: Leveraging AI to tackle global challenges, such as climate change, poverty, and healthcare disparities.
- Human-AI Collaboration: Exploring new ways for humans and AI systems to work together, augmenting human capabilities and enabling new forms of creativity and innovation.
What is the difference between AI, ML, and deep learning?
+AI is the broader concept of creating machines that can perform tasks requiring human-like intelligence. ML is a subset of AI, focusing on algorithms that can learn from data. Deep learning is a type of ML that uses neural networks with multiple layers to learn complex representations.
Can AI systems be biased?
+Yes, AI systems can exhibit bias if the training data or algorithms contain inherent biases. This can lead to unfair or discriminatory outcomes, highlighting the importance of addressing bias and fairness in AI development.
How can AI be used to address climate change?
+AI can be applied to various aspects of climate change, such as predicting extreme weather events, optimizing energy consumption, and monitoring deforestation. For example, AI-powered sensors can detect changes in forest cover, enabling timely interventions to prevent further damage.
What are the ethical considerations in AI development?
+Ethical considerations in AI development include ensuring transparency, accountability, and fairness in AI systems. Developers must also address issues related to privacy, security, and the potential impact of AI on employment and society.
In conclusion, the evolution of AI has been a remarkable journey, marked by significant advancements and challenges. As we look to the future, it’s essential to prioritize responsible AI development, addressing ethical concerns and ensuring that AI benefits humanity as a whole. By doing so, we can unlock the full potential of AI and create a more equitable, sustainable, and prosperous world.